Special Session XV
Intelligent Fault Diagnosis with Target Data Scarcity
Submission Guideline:
Please submit your manuscript via Online Submission System: https://easychair.org/conferences/?conf=rcae2024
Please choose Special Session: Intelligent Fault Diagnosis with Target Data Scarcity
Introduction:
With the booming development of the information science and the recent artificial intelligence, many intelligent fault diagnosis (IFD) methods incorporating deep learning have been extensively investigated and achieved remarkable success. However, these methods heavily rely on large-scale identically distributed labeled data. In reality, this assumption is often difficult to satisfy due to the scarcity of fault samples and the variability and complexity of working conditions. This session aims to explore the latest developments and application technologies of IFD methods with scarce label data.
Topics of interest include, but not limited to:
1. Zero-shot/ few-shot based IFD methods
2. Transfer learning based IFD methods
3. Physics-guided neural network based IFD methods
4. Decentralized federated learning based IFD methods
5. Combined model-based and data-driven IFD methods
6. Advanced class-imbalanced learning based IFD methods
Organizers:
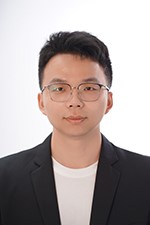
Shixiang Lu, Associate Professor
Northeast Petroleum University, China
Shixiang Lu received the B.Sc. degree from the Hefei University of Technology (HFUT), Hefei, China, and the Ph.D. degree in management science and engineering from HFUT in 2023. He was a visiting Ph.D. student at the Faculty of Engineering and Environment, University of Northumbria, U.K during 2021-2023. His research interests include smart manufacturing, computational intelligence applications in fault diagnosis and prognosis.
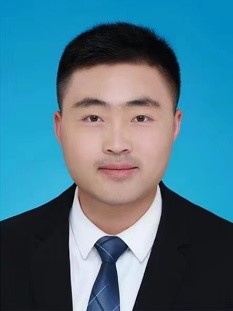
Hailang Jin, Postdoctor
Tsinghua University, China
Hailang Jin received the B.E. degree in measurement and control technology and instrument from Shenyang University of Technology, Liaoyang, China, in 2016, the M.E. degree in control engineering from Guangxi University, Nanning, China, in 2018 and the Ph.D. degrees in control science and engineering from Tianjin University, Tianjin, China, in 2024. From November 2021 to November 2022, he was a visiting Ph.D. student sponsored by China Scholarship Council at the Faculty of Engineering and Environment, University of Northumbria, Newcastle upon Tyne, U.K. Now he is a postdoctoral research fellow with the Department of Automation, Tsinghua University. His research interests include fault diagnosis and fault-tolerant control, robust control, adaptive control.
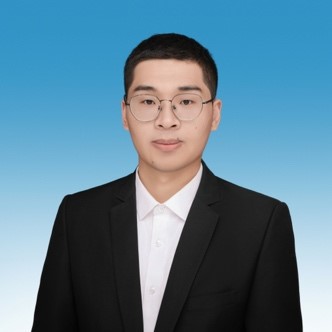
Chuang Wang, Postdoctor
Northeast Petroleum University, China
Chuang Wang received the B.Sc. degree in automation and the Ph.D. degree in petroleum and natural gas engineering from the Northeast Petroleum University, Daqing, China, in 2017 and 2023, respectively. From 2022 to 2023, he was a Visiting Ph.D. Student with the Department of Computer Science, Brunel University London, Uxbridge, U.K. He is currently a Post-Doctoral Research Fellow with the Artificial Intelligence Energy Research Institute, Northeast Petroleum University, Daqing, China. His research interests include evolutionary computation and transfer learning applications in fault diagnosis.
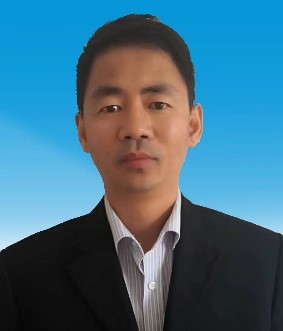
Yuanhong Liu, Associate Professor
Northeast Petroleum University, China
Yuanhong Liu received the Ph.D. degrees in control science and engineering from Harbin Institute of Technology, Harbin, China, in 2016. From 2018 to 2019, he was a Visiting scholar with the Faculty of Engineering and Environment, University of Northumbria, U.K. Now he is an associate professor with the School of Electrical Engineering & Information Science and Engineering, Northeast Petroleum University. His research interests include manifold learning, data-driven and artificial-intelligence based fault diagnosis, Intelligent Control.
- Home
- Call for Papers
- Call for Special Session
- Special Session I
- Special Session II
- Special Session III
- Special Session IV
- Special Session V
- Special Session VI
- Special Session VII
- Special Session VIII
- Special Session IX
- Special Session X
- Special Session XI
- Special Session XII
- Special Session XIII
- Special Session XIV
- Special Session XV
- Special Session XVI
- About Conference
- For Attendees
- History
- Contact
- 中文