Special Session XVI
Data-driven Modeling and Optimization for Large-scale Systems in Intelligent Manufacturing
Submission Guideline:
Please submit your manuscript via Online Submission System: https://easychair.org/conferences/?conf=rcae2024
Please choose Special Session: Data-driven Modeling and Optimization for Large-scale Systems in Intelligent Manufacturing
Introduction:
Data-driven modeling and optimization techniques play an important role in large-scale industrial systems central to intelligent manufacturing. By applying advanced analytics, machine learning algorithms, and artificial intelligence, manufacturers can predict system behaviors, optimize operational parameters, and achieve significant improvements in productivity and quality. This leads to smarter, more flexible, and sustainable manufacturing systems equipped to meet the challenges of the modern industrial landscape. However, there are several key challenges. First, the data collected from large-scale industrial systems exhibit dynamic, high-dimensional, and nonlinear characteristics, which pose significant difficulties for effective feature extraction using existing methods. Second, the complexity of neural networks results in a lack of interpretability in deep learning methods. Therefore, this special session calls for interpretable designs for data-driven modeling and intelligence optimization.
Any interpretable enhancement technique for large-scale industrial system modeling falls within this scope, including, but not limited to, deep learning methods, data-driven modeling, performance interpretability analysis, etc.
Organizers:
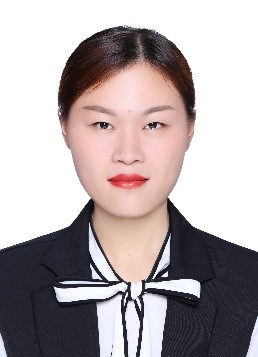
Xiuli Zhu, Lecturer
University of Shanghai for Science and Technology, China
Xiuli Zhu received the Ph.D. degree in control science and engineering from Donghua University, Shanghai. She was a Visiting Scholar with the Department of Chemical and Materials Engineering, University of Alberta, Edmonton, AB, Canada, from September 2019 to September 2021. She is currently a Lecturer at the University of Shanghai for Science and Technology, Shanghai, China. Her research interests include explainable artificial intelligence, fault diagnosis, data-driven modeling, and intelligence optimization.
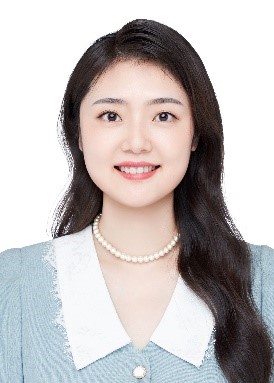
Ling Li, Lecturer
Changsha University of Science & Technology, China
Ling Li received her Ph.D. degree from the School of Automation, Central South University, Changsha, China, in 2020. She was a visiting scholar with the Department of Chemical and Materials Engineering, University of Alberta, Edmonton, AB, Canada, from December 2019 to June 2020. She is currently a Lecturer at the School of Electrical & Information Engineering, Changsha University of Science & Technology. Her research interests include deep learning and machine learning, process operating performance assessment, process data analysis, etc.
- Home
- Call for Papers
- Call for Special Session
- Special Session I
- Special Session II
- Special Session III
- Special Session IV
- Special Session V
- Special Session VI
- Special Session VII
- Special Session VIII
- Special Session IX
- Special Session X
- Special Session XI
- Special Session XII
- Special Session XIII
- Special Session XIV
- Special Session XV
- Special Session XVI
- About Conference
- For Attendees
- History
- Contact
- 中文